Human beings often tend to feel invincible. We think that nothing should come in the way of the life we envision for ourselves. But all it took was one microscopic virus to change the global world and how it functions. These days, people wearing masks and covering their faces with shields has become a common sight. The coronavirus pandemic has sparked global concern, and we are now left scrambling for a solution.
Before we delve into the role of big data analytics in mitigating a pandemic, let us look at some worldwide attempts to control the coronavirus. In the USA, we notice a stark leadership vacuum – the anti-mask stance of Trump, and his prioritisation of the economy over human health, has led to skyrocketing infectivity rates. Coupled with this, the World Health Organisation was initially denying that the coronavirus was a pandemic! In India, despite efficient government interventions – a timely lockdown, and even turning stadiums into Covid care units – it is the lack of public health awareness among the masses responsible for the spread of the virus. Hence, the judgements of human beings, the governments, and even notable organisations, cannot seem to be trusted to deal with a pandemic which requires swift and unbiased action. It is safe to say that they are too fragile to deal with a crisis of this magnitude, and some of them may even serve a vested interest, rather than having an international mindset for the greater good. Therefore, we need to defend ourselves from the pandemic by relying on a much more unbiased, neutral, and reliable source. According to me, the solution lies in big data analytics.
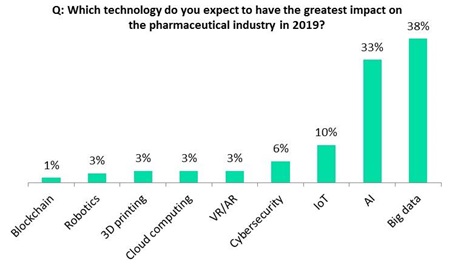
Fig 1: The use of Big Data and Artificial Intelligence already contributes a whopping 70% to the pharma industry and is only set to grow in the field of future healthcare practises.
There are advantages and disadvantages of using big data but it is certainly more dependable than human judgement. Artificial intelligence and machine learning techniques form the basis of predictive modeling of disease, which can track the spread of any microbe. In the future, an important scientific model for safe healthcare could be the development of an integrative control strategy involving contact tracing and developing route maps of infected patients which will help predict potential disease hotspots. Imagine a real-time update of vacancy in hospital beds along with such route maps. When hospitals themselves have become a hotbed for contracting Covid, the creation of a smartphone app providing dynamic updates of doctor availability and hospital bed vacancy, would reduce the rush of patients and create a safer environment for healthcare. In India, patients are dying on the way to hospitals, after being refused multiple times due to lack of beds. Big data can be used to help us conduct a quick search where we can be notified of hospital bed vacancies from our homes itself, for not only a pandemic but any disease, saving crucial travel time and costs for patients. This can be expanded to include important details such as wait time for admission into the emergency ward, especially required during accidents. In the future, we can hope for publicly visible clinical dashboards that will showcase real-time data regarding testing results for any health crises, including patient load, and available nursing units. This is vital for the growth of a robust healthcare system.
Big data is also of vital importance for scientists studying the virus and attempting to find a vaccine. Vaccines are designed by running simulations of drug-virus interactions based on the study of previously available data regarding behaviour of micro – organisms, which help determine the efficacy of the vaccine. These datasets are plotted as graphs which facilitate analysis of the trends and patterns of viral behaviour. These minute data help to process greater scientific models, and only based on big data analytics can vaccines be declared fit for human clinical trials. Artificial intelligence, combined with big data analytics, can be used to make appropriate predictions for any such disease outbreak in the future.
It is evident that the data we collect from the pandemic now, is our strongest weapon to deal with similar crises in the future. This has been effectively demonstrated by a country such as Taiwan, which had harnessed big data and machine learning tools after the 2003 SARS outbreak, to predict the spread of disease and to minimise the effect on its territory. Despite Taiwan being at such close proximity to China, located at a mere distance of 130 kilometres, it managed to prevent a severe outbreak by integrating the database of its national health insurance with data from immigration, air travel, and customs, thus facilitating the tracking of clinical visits based on travel history. Effective use of Quick Response (QR) codes scanned at airports also helped to create a national database with details of travel history of symptomatic passengers, who were later monitored via location trackers in smartphones, to help ensure strict adherence to self quarantine and isolation protocol during incubation periods. Advanced scientific study of data based on SARS was also of great help to the frontline warriors who were competently trained enough to fight the pandemic. The Taiwan success story is testament to the fact that the future of a strong healthcare system lies in data modelling learnt as a lesson from the pandemic we are dealing with today.
New Zealand and South Korea have also proved how big data is the key to controlling the pandemic. The government of South Korea systematically updated its citizens about the route maps of contacts of each new confirmed case, so that they could avoid coming in contact with contaminated zones. New Zealand, the first country to be publicly recognised as coronavirus-free, communicated disease modelling data to policy makers to ensure the formulation of a scientific approach to testing and treatment.
Therefore, I believe that it is only the effective harnessing of big data – ranging from predictive modeling to vaccine development – which can pave the right path for the future by creating safe healthcare practices. But this cannot be an effort by the data science community alone. It needs an interdisciplinary approach backed by the support of healthcare experts as well as skilled biomedical workers. Public trust and cooperation is also of paramount importance, especially in the context of data privacy issues. In addition to this, there will always be missing data variables that will prevent us from seeing the complete picture. But, if the healthcare workers, data scientists, and epidemiologists come together, we can harness the big data revolution to our benefit.
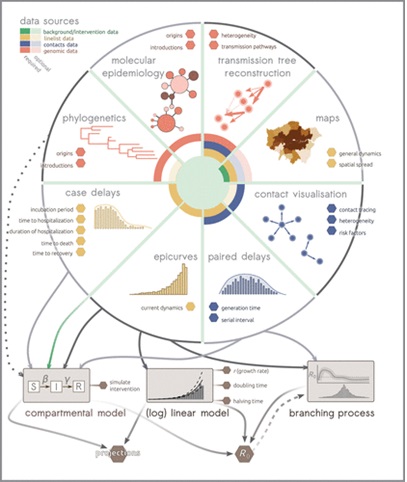
Fig 2: ‘Outbreak analysis’ as defined in a study conducted by The Royal Society uses developing data science to analyse various parameters (contact data, genomic data etc) involved in controlling a pandemic.
The world is in the midst of the fourth industrial revolution of digital transformation, and the future will be more data driven than ever before. Data science has evolved to the point where we can predict the expected path of a natural disaster such as a cyclone – as the state of West Bengal accurately did for cyclone Amphan of 2020, and started prior evacuation in areas projected to be worst hit, thereby saving millions of lives in advance. Imagine, in the future, if we could predict the path of a pandemic, by tracing it from the advent of the outbreak, and restrict it to remain an epidemic, preventing the progress of the disease. If international travel history data from one coronavirus epicentre to another was harnessed in such projection, perhaps countries such as Italy, caught off guard by the sudden force of the coronavirus, would not lose their helpless citizens and frontline workers trapped in an underprepared and overburdened healthcare system. Similar predictive models in the future can even be applied in medical imaging of a novel disease, and data collection based on individual patients will help devise more precise and personalised treatment. This is especially relevant given that the coronavirus has a set of evolving symptoms rather than fixed parameters for diagnosis – these multiple symptoms were uncovered by processing a multitude of raw data, and perhaps a larger and more diverse database can unveil more updated biomarkers, which will help us assess our response to the disease more accurately. Big data is not only an important part of scientific modeling in future healthcare, big data itself is the future – hiding within masses of data lies information that can change the world.
————————————————————————————————————————–
BIBLIOGRAPHY
- Mehta, A. (2020, May 22). Big Data and COVID-19: Proceed with Caution. Retrieved August 26, 2020, from https://www.outlookindia.com/website/story/opinion-big-data- has-potential-to- save-lives-in-covid-times-but-proceed-with-caution/353390
- Marr, B. (2020, April 09). The Vital Role Of Big Data In The Fight Against Coronavirus. Retrieved August 26, 2020, from https://www.forbes.com/sites/bernardmarr /2020/04/ 09/the-vital-role-of-big-data-in-the-fight-against-coronavirus/
- Jones, A. (2020, July 10). How did New Zealand become Covid-19 free? Retrieved August 26, 2020, from https://www.bbc.com/news/world-asia-53274085
- S, R., Here, P., -, K., -, D., & -, A. (2020, June 01). ICMR data shows India’s Covid testing is not in right shape – 5 things that need fixing. Retrieved August 26, 2020, from https://theprint.in/opinion/icmr-data-shows-indias-covid-testing-is-not-in-right- shape-5-things-that-need-fixing/433077/
- Healthcare, G. (2018, December 20). Big Data and AI will drive innovation, revenue and operational efficiencies in 2019. Retrieved August 30, 2020, from https://www.pharmaceutical-technology.com/comment/big-data-and-ai-will-drive-innovation-revenue-and-operational-efficiencies-in-2019/
- A., P., Jonathan A. Polonsky Jonathan A. Polonsky http://orcid.org/0000-0002-8634-4255 Department of Health Emergency Information and Risk Assessment, Polonsky, J., Jonathan A. Polonsky http://orcid.org/0000-0002-8634-4255 Department of Health Emergency Information and Risk Assessment, Baidjoe, A., Amrish Baidjoe http://orcid.org/0000-0001-5295-5085 Department of Infectious Disease Epidemiology, . . . Al., E. (2019, May 20). Outbreak analytics: A developing data science for informing the response to emerging pathogens. Retrieved August 30, 2020, from https://royalsocietypublishing.org/doi/10.1098/rstb.2018.0276